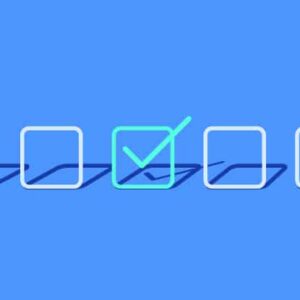
Survey Vs Focus Group
Survey Vs Focus Group Voxco is trusted by 450+ Global Brands in 40+ countries See what question types are possible with a sample survey! Try
Find the best survey software for you!
(Along with a checklist to compare platforms)
Take a peek at our powerful survey features to design surveys that scale discoveries.
Explore Voxco
Need to map Voxco’s features & offerings? We can help!
Find the best customer experience platform
Uncover customer pain points, analyze feedback and run successful CX programs with the best CX platform for your team.
We’ve been avid users of the Voxco platform now for over 20 years. It gives us the flexibility to routinely enhance our survey toolkit and provides our clients with a more robust dataset and story to tell their clients.
Steve Male
VP Innovation & Strategic Partnerships, The Logit Group
Explore Regional Offices
Find the best survey software for you!
(Along with a checklist to compare platforms)
Take a peek at our powerful survey features to design surveys that scale discoveries.
Explore Voxco
Need to map Voxco’s features & offerings? We can help!
Find the best customer experience platform
Uncover customer pain points, analyze feedback and run successful CX programs with the best CX platform for your team.
We’ve been avid users of the Voxco platform now for over 20 years. It gives us the flexibility to routinely enhance our survey toolkit and provides our clients with a more robust dataset and story to tell their clients.
Steve Male
VP Innovation & Strategic Partnerships, The Logit Group
Explore Regional Offices
Find the best survey software for you!
(Along with a checklist to compare platforms)
Take a peek at our powerful survey features to design surveys that scale discoveries.
Explore Voxco
Need to map Voxco’s features & offerings? We can help!
Find the best customer experience platform
Uncover customer pain points, analyze feedback and run successful CX programs with the best CX platform for your team.
We’ve been avid users of the Voxco platform now for over 20 years. It gives us the flexibility to routinely enhance our survey toolkit and provides our clients with a more robust dataset and story to tell their clients.
Steve Male
VP Innovation & Strategic Partnerships, The Logit Group
Explore Regional Offices
SHARE THE ARTICLE ON
When we look at data and research, it’s important to know the difference between correlation and causation. These are two big words that mean different things but are often mixed up. In this blog post, we’ll talk about what they mean, give examples, and explain why knowing the difference is so important.
Embark on your journey towards data-driven success!
In analytics, correlation shows a connection between variables. When one changes, the other follows suit. It is important to understand that this connection doesn’t always suggest a direct or indirect cause between the variables. It’s important to understand that the relationship between variables doesn’t always mean one causes the other.
there are three main types of correlations between variables:
1. Positive Correlation: When variables have a positive correlation, it means they move in the same direction. When x increases, y increases, and when x decreases, y decreases.
2. Negative Correlation: When variables have a negative correlation, it means they move in opposite directions. When x increases, y decreases, and when x decreases, y increases.
3. No Correlation: When variables have no correlation, it means there is no relationship between them. When x increases, y either stays the same or has no distinct pattern.
Take, for instance, the following example: When temperatures rise in the summer, both ice cream sales and swimsuit sales increase. Despite the positive correlation between ice cream and swimsuit sales, there’s no causal link between these variables. Rather, the rise in temperature separately influences both of these variables, resulting in their positive correlation.
In analytics, causation refers to a relationship where one variable is dependent on the other, indicating a causal relationship. In a causal relationship, there is an independent variable (‘the cause’) and a dependent variable (‘the effect’).
Let’s continue with the aforementioned example:
We’ve established that ice cream and swimsuit sales correlate positively in the summer due to temperature rises. In this example, temperature has a causal relationship with both ice cream and swimsuit sales separately. When the temperature rises, more people purchase ice creams; when the temperature rises, more people purchase swimsuits.
It’s crucial to recognize that correlation does not imply causation. While correlated variables may exhibit similar patterns, correlation alone does not establish a causal relationship. The key differences between correlation and causation include:
Reflecting on the initial statement: variables with a causal relationship are indeed related, meaning causation always implies correlation. However, as variables can be related without directly influencing each other, correlation does not imply causation
Get Market Research Trends Guide, Online Surveys Guide, Agile Market Research Guide & 5 Market Research Template
Let’s understand a few reasons why we cannot imply causation even if we identify correlation:
In exploring this disparity, it’s crucial to consider concepts like linear relationships and randomized, controlled experimental designs. For instance, conducting controlled experiments to collect data on the sale of ice cream can help elucidate the causal relationship between temperature and ice cream sales.
When determining if your product causes a particular outcome or behavior, it’s crucial to test it rigorously and precisely.
Here are some steps you can take to test for causation:
Start by clearly defining the hypothesis you want to test. What specific effect do you believe your product has on users? For example, if you’re testing a new fitness app, your hypothesis might be that using the app leads to an increase in daily exercise.
Create controlled experiments to isolate the effect of your product from other variables. This might involve dividing users into different groups, such as a treatment group that uses your product and a control group that does not. Ensure that the groups are similar in all other aspects except for the presence of your product.
Identify key metrics that reflect the desired outcome of your product. These could include changes in user behavior, attitudes, or performance indicators. Use reliable measurement tools to track these metrics accurately over time.
Use statistical methods and analysis techniques that allow you to draw causal conclusions from your data. Seek evidence that shows a causal relationship between your product’s usage and the observed data in the measured metrics. Be cautious of confounding variables, and make sure that your product is the clear cause of any observed effects.
Evaluate alternative explanations for the observed results. Could factors other than your product be influencing the outcome? Account for potential confounding variables and ensure they are controlled for in your analysis.
Repeat your experiments to validate your results and ensure their reliability. Replication increases confidence in the causal relationship between your product and the observed effects.
By following these steps, you can gain valuable insights into the causal impact of your product on user behavior and outcomes. This information can inform product development, marketing strategies, and decision-making processes, ultimately leading to more effective and successful products.
Comprehending the disparity between correlation and causation is paramount across diverse arenas, encompassing science, economics, and public policy. While correlation unveils associations between variables, causation elucidates the mechanisms propelling those associations. By discriminating between the two, researchers and decision-makers can formulate more precise conclusions and craft informed decisions.
Curious About The Price? Click Below To Get A Personalized Quote.
What does ‘correlation does not imply causation’ mean?
A few reasons why correlation may not imply causation are;
What is a correlation?
A correlation defines a statistical association between variables.
What is causation?
Causation suggests that altering one variable triggers a change in the other, demonstrating a causal connection between them.
What is the difference between correlation and causation?
It’s crucial to recognize that correlation does not imply causation. While correlated variables may exhibit similar patterns, correlation alone does not establish a causal relationship. The key differences between correlation and causation include:
Survey Vs Focus Group Voxco is trusted by 450+ Global Brands in 40+ countries See what question types are possible with a sample survey! Try
How to Write a Research Methodology SHARE THE ARTICLE ON Table of Contents What is a Research Methodology? A research methodology is an outline of
Brand Positioning Statement Examples: Crafting Your Brand’s Essence SHARE THE ARTICLE ON Table of Contents To thrive in a competitive business landscape, you need to
How to use Multiple Choice Questions in your survey? SHARE THE ARTICLE ON See what question types are possible with a sample survey! Try a
Online Survey Analysis Tool: Tips and Best Practices SHARE THE ARTICLE ON Table of Contents Data are an integral part of making informed decisions in
All You Need To Know About Survey Translation SHARE THE ARTICLE ON Table of Contents In this increasingly interconnected and globalized world, where customers can
We use cookies in our website to give you the best browsing experience and to tailor advertising. By continuing to use our website, you give us consent to the use of cookies. Read More
Name | Domain | Purpose | Expiry | Type |
---|---|---|---|---|
hubspotutk | www.voxco.com | HubSpot functional cookie. | 1 year | HTTP |
lhc_dir_locale | amplifyreach.com | --- | 52 years | --- |
lhc_dirclass | amplifyreach.com | --- | 52 years | --- |
Name | Domain | Purpose | Expiry | Type |
---|---|---|---|---|
_fbp | www.voxco.com | Facebook Pixel advertising first-party cookie | 3 months | HTTP |
__hstc | www.voxco.com | Hubspot marketing platform cookie. | 1 year | HTTP |
__hssrc | www.voxco.com | Hubspot marketing platform cookie. | 52 years | HTTP |
__hssc | www.voxco.com | Hubspot marketing platform cookie. | Session | HTTP |
Name | Domain | Purpose | Expiry | Type |
---|---|---|---|---|
_gid | www.voxco.com | Google Universal Analytics short-time unique user tracking identifier. | 1 days | HTTP |
MUID | bing.com | Microsoft User Identifier tracking cookie used by Bing Ads. | 1 year | HTTP |
MR | bat.bing.com | Microsoft User Identifier tracking cookie used by Bing Ads. | 7 days | HTTP |
IDE | doubleclick.net | Google advertising cookie used for user tracking and ad targeting purposes. | 2 years | HTTP |
_vwo_uuid_v2 | www.voxco.com | Generic Visual Website Optimizer (VWO) user tracking cookie. | 1 year | HTTP |
_vis_opt_s | www.voxco.com | Generic Visual Website Optimizer (VWO) user tracking cookie that detects if the user is new or returning to a particular campaign. | 3 months | HTTP |
_vis_opt_test_cookie | www.voxco.com | A session (temporary) cookie used by Generic Visual Website Optimizer (VWO) to detect if the cookies are enabled on the browser of the user or not. | 52 years | HTTP |
_ga | www.voxco.com | Google Universal Analytics long-time unique user tracking identifier. | 2 years | HTTP |
_uetsid | www.voxco.com | Microsoft Bing Ads Universal Event Tracking (UET) tracking cookie. | 1 days | HTTP |
vuid | vimeo.com | Vimeo tracking cookie | 2 years | HTTP |
Name | Domain | Purpose | Expiry | Type |
---|---|---|---|---|
__cf_bm | hubspot.com | Generic CloudFlare functional cookie. | Session | HTTP |
Name | Domain | Purpose | Expiry | Type |
---|---|---|---|---|
_gcl_au | www.voxco.com | --- | 3 months | --- |
_gat_gtag_UA_3262734_1 | www.voxco.com | --- | Session | --- |
_clck | www.voxco.com | --- | 1 year | --- |
_ga_HNFQQ528PZ | www.voxco.com | --- | 2 years | --- |
_clsk | www.voxco.com | --- | 1 days | --- |
visitor_id18452 | pardot.com | --- | 10 years | --- |
visitor_id18452-hash | pardot.com | --- | 10 years | --- |
lpv18452 | pi.pardot.com | --- | Session | --- |
lhc_per | www.voxco.com | --- | 6 months | --- |
_uetvid | www.voxco.com | --- | 1 year | --- |