The Voxco Answers Anything Blog
Read on for more in-depth content on the topics that matter and shape the world of research.
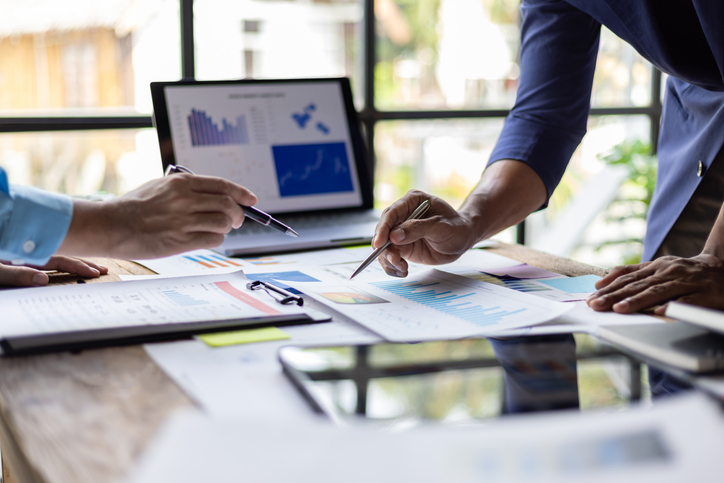
The Latest in Market Research
Marketing Research vs. User Research: An Unnecessary Divide?
With separate associations, conferences, and certifications for marketing researchers and user experience researchers, the two are often treated as distinct disciplines. While there are some differences, are we splitting hairs and creating an unnecessary and unwelcome divide? Let’s have a look at how much marketing researchers and user researchers have in common and how we can learn from each other.
Both seek to understand human behavior
At their core, both marketing researchers and user researchers seek to understand consumer and customer behaviors and preferences to drive better quality business decision-making. Where marketing researchers are trying to understand brand and product preferences and UX researchers are trying to understand how people want to experience and interact with those products and services, the underlying questions remain the same.
What do people want, need, and struggle with? When, where, why, and how are they struggling with those things? What is the human experience and how can we understand that experience more deeply and accurately?
Both UX researchers and market researchers have a genuine interest in the human experience and want to understand people.
Both share a methodological toolbox
Whether you have a personal preference for qualitative or quantitative techniques, marketing researchers and user researchers pull from the same toolbox overflowing with qualitative, quantitative, experimental, and correlational techniques. For example:
- Quantitative surveys: Both market researchers and UX researchers rely heavily on surveys. The quantitative data gathered from surveys helps both groups gather standardized data to describe differences among groups in unbiased ways and track trends over time. Surveys are an excellent way to quantify perspectives of both user experiences and brand perceptions.
- Interviews and focus groups: Focus groups and interviews are also heavily used by both types of researchers. Often, the best way to understand concerns and problems is to have a personal conversation with a researcher who is trained in thoughtful probing. Whether the thing being discussed is the UX of a website or product package, or the strengths and weaknesses of a TV commercial or a community program, inviting people to talk through their perceptions with a researcher, whether online or face to face, elicits deep and very personal insights.
- A/B and Test/Control designs: Whether it’s called A/B testing or Test/Control research, at the heart of much research is experimental design. The direct and controlled comparison of one group to another group can help us to understand which user experience or brand personality is preferred.
The same goes for shop-alongs, analytics, biometrics, bulletin-boards, AI/chatbot interviewing, and a host of other research techniques. All are well-loved and well-used by both marketing and user researchers.
Divergent goals and scope
So far, marketing and UX research have a lot in common. The key difference, however, lies in their goals and where their expertise is applied in the lifecycle of a product or service.
Marketing researchers embrace a broad range of goals over the entire product lifecycle. From pre-development innovations to pricing, customer experience, customer journeys, and market opportunities, their outputs are used extensively by brand managers, business strategists, and sales teams to solve a wide range of business problems.
On the other hand, user researchers have more narrow goals that focus on one aspect of the product lifecycle. Specifically, they seek to understand how people experience and interact with products and services, insights that are used not only by brand managers, business strategists, and sales teams, but also by product designers and engineers.
Marketing researchers address a wide range of objectives including UX research whereas UX researchers specialize in and have extensive experience in only UX research.
What can marketing researchers learn from user researchers?
Whether we’re marketing or user researchers, all researchers have generalist skills. We’re familiar with the pros and cons of questionnaire, focus group, interview, bulletin-board, shop-along, and analytics research tools. We’re familiar with considerations related to ethics, privacy, culture, and community nuances. We may not know the intricate details of every aspect but we know enough to advise research buyers on which technique would best suit their purposes and how to choose the best supplier to meet their needs.
Similarly, most researchers have specialist skills, expertise, and preferences. UX researchers love figuring out, understanding, and improving the user experience. Similarly, some marketing researchers love creating personal connections with consumers via focus groups and interviews while others love pricing research and the fun that goes along with conjoint analysis, Van Westendorp’s Price Sensitivity Meter, and the Gabor-Granger technique.
Perhaps the key difference between marketing researchers and UX researchers is that user researchers have whole-heartedly embraced being specialist researchers with niche skills. Is it time for marketing researchers to take this as a cue? Many of us aren’t generalist qualitative researchers or quantitative researchers. We’re brand equity researchers, pricing researchers, packaging researchers, or persona researchers.
Depending on our unique passions, we too could champion and identify ourselves in these ways. Just as brand managers always know who to turn to when they need UX research, let’s help them know who to turn to when they need journey mapping research, package testing research, or ad testing research. We might even encourage our research associations to develop subject matter certifications for pricing researchers, brand equity researchers, and loyalty researchers.
For those of you who are already specialist researchers or who have chosen a path to become experts in online surveys, CATI, or qualitative coding, please get in touch with one of our experts. We’d love to be part of your expert team!
Read more

How to Choose the Right Solution
How a Global Beauty Retailer Used In-House Research to Stay Ahead in a $600B Market
In beauty and personal care, yesterday’s trend can become today’s shelf-warmer. To stay ahead, one global retailer—operating across 35+ countries—decided to rethink how they collected and used customer insight.
Instead of chasing trends, they built a system to lead them.
The Challenge: When the Market Won’t Wait
The global beauty market hit $446B in 2023—and it’s still growing. Competing in this space requires more than great products. It requires real-time understanding of what customers want, how they shop, and what drives them to return.
This brand knew they needed to move faster, test smarter, and act with confidence. That meant bringing research in-house—with the right tools to back them up.
The Strategy: Make Insight Part of Every Decision
With the right tools, the brand was able to build an agile research system that supported smarter, faster decisions across the business.
Key pillars of the strategy:
- Comprehensive product and service testing
Every new concept—from skincare lines to loyalty features—was tested for appeal and purchase potential before launch using Voxco’s survey tools. Only the most promising ideas made it to market.
- Custom-built brand indicators
The team developed internal barometers—like a proprietary “attractive brand” score—to track performance and customer sentiment over time.
- Real-time access across teams
Insights didn’t sit in dashboards—they were shared across departments to inform marketing, merchandising, and operations in real time.
The Results: Scalable, Cost-Efficient Research That Delivers
By managing consumer research internally with Voxco, the retailer gained three core advantages:
- Agility
Surveys could be designed, launched, and iterated quickly—helping the brand respond to shifting trends without delay.
- Cost-efficiency at scale
In-house execution meant more research could be done, more often, without the overhead of outsourcing.
- Trustworthy, decision-ready data
With direct control over survey design and data quality, every insight was dependable—and immediately usable.
The Ripple Effect: Insight That Powers the Whole Business
The benefits extended far beyond product teams. Inventory managers used trend data to optimize stock levels. Store operators improved frontline service with customer experience feedback. Brand managers refined campaigns based on real-time sentiment.
Want to learn how you can build a scalable, insight-led strategy that drives decisions across teams? Book a demo with Voxco to see it in action.
Read more

The Latest in Market Research
Text Analytics & AI
Why Coders in Market Research Shouldn’t Fear AI
If you work in market research coding—specifically with open-ended responses—you’ve likely heard the chatter: “AI can do it now.” With the rise of tools that can automatically classify free-text comments, summarize themes, and even simulate human responses, it's easy to worry that your role might be on the chopping block.
But here’s the reality: while AI is changing how open-ended coding is done, it’s not replacing the need for skilled human coders. In fact, your expertise is more important than ever.
Let’s talk about why.
1. AI Still Needs Human Oversight
Yes, AI can classify open-ended comments, but it’s far from perfect. It misses nuance, struggles with sarcasm, fails to understand context, and often makes inconsistent calls. If you’ve ever audited machine-coded responses, you know: it still takes a human eye to ensure quality.
Human coders bring judgment—a sense of tone, relevance, and deeper meaning—that AI just can’t replicate reliably.
2. Category Schemes Don’t Build Themselves
Before any AI can classify text, someone needs to define the coding frame—the categories, definitions, and boundaries for what goes where. And when the data shifts (new product, new market, new audience), that structure needs adjusting.
Creating and refining these frameworks is a creative and analytical task. It takes market knowledge, business understanding, and the ability to connect dots between consumer language and client objectives. That’s your domain.
3. Clients Still Care About the "Why"
Clients don’t just want a dashboard of tags. They want to understand what people mean, how they feel, and what drives their behavior. Open-ended responses are where that gold lives. AI can help speed things up—but humans are still needed to interpret, synthesize, and communicate insights.
What does “It just feels cheap” really mean in a brand perception study? Is it about price, quality, packaging, or social status? That kind of insight doesn’t come from a model—it comes from you.
4. Edge Cases Matter More Than You Think
In market research, it’s often the outliers—the odd comments, unexpected complaints, or surprising sentiments—that lead to meaningful discoveries. AI tends to smooth those over or misfile them entirely. Human coders spot the oddities, dig deeper, and surface insights that algorithms overlook.
You're not just categorizing data. You're finding what matters.
5. You’re Becoming a Strategist, Not Just a Coder
The role of a coder is evolving. It’s moving from manual labeling to quality control, code-frame design, model training, and insight generation. This is good news: it means your work is becoming more strategic, not less.
If you're adapting and learning how to work with AI—auditing its output, guiding its accuracy, and integrating it into your workflow—you’re positioning yourself as a core part of the insights process, not a casualty of automation.
Final Thoughts
The future of open-ended coding isn’t about choosing between humans and machines—it’s about combining strengths. AI can handle the bulk; you bring the brain.
So no, your job isn’t disappearing. It’s evolving. And if you’ve built your skills around critical thinking, pattern recognition, and insight generation, you’re not just safe—you’re essential.
Read more
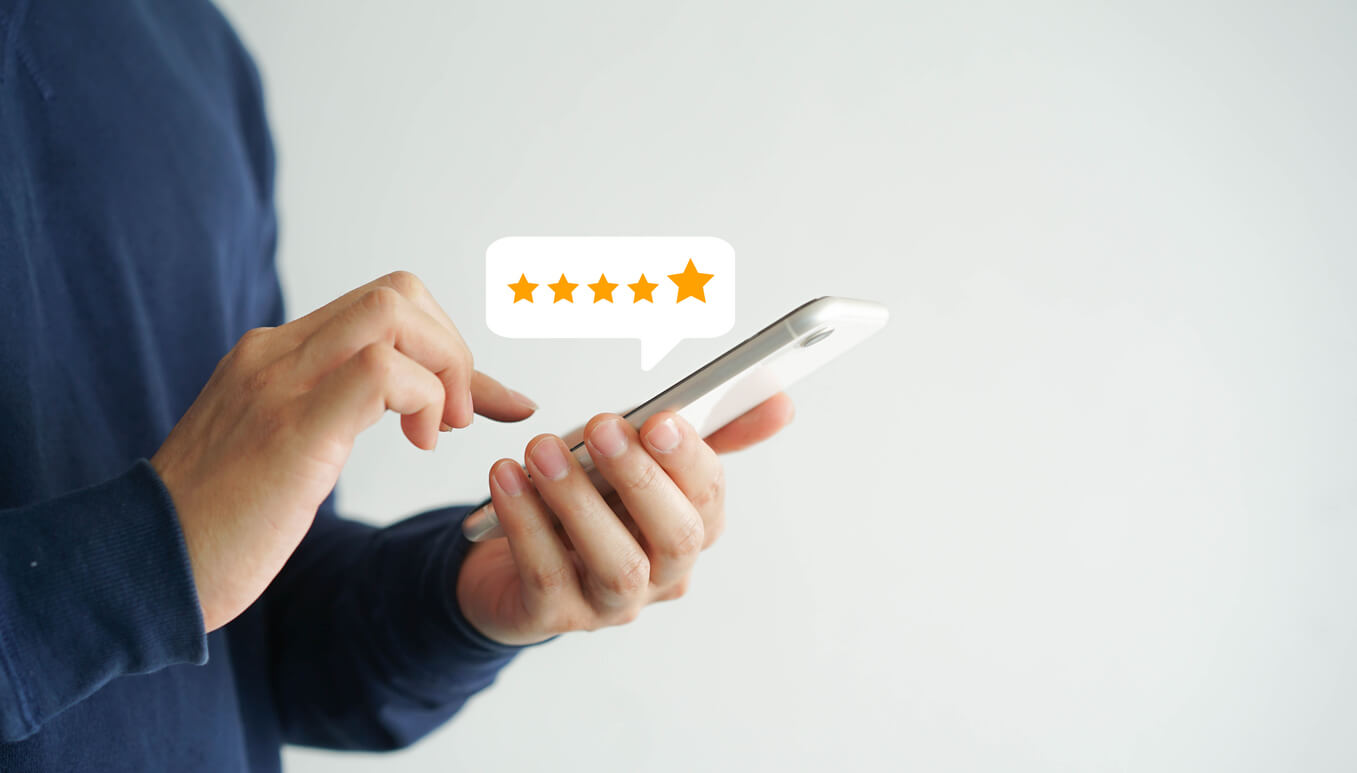
Market Research 101
The Latest in Market Research
Customer Metrics That Matter: From CSAT to CX to Customer Success
Customer-centric strategies are critical drivers of long-term business success. They drive revenue, competitive advantage, innovation, and they future-proof the business. To become customer-centric, businesses need to understand the differences and connections among three key concepts: Customer experience (CX), customer satisfaction (CSAT), and customer success.
Customer Satisfaction (CSAT) as a Discrete Experience
The last time you purchased something, you followed a process of visiting one or more physical or digital retail outlets, reviewing the options, and selecting the product or service that best met your needs. In the moment, you felt some degree of satisfaction with the website you used, the employee who helped you, or the packaging you handled. This is the scenario in which measuring CSAT is most applicable – your satisfaction with the specific touchpoints you encountered on that single occasion.
Measuring customer satisfaction serves many purposes. It helps business leaders, marketers, and innovators identify pain points at specific points in the customer journey, issues with products and services requiring improvements, and gaps that can be filled by creating new products and services.
The most common research technique for measuring CSAT is the ubiquitous survey. Airport security lines and shopping mall bathrooms present digital devices with red or a green buttons to their clientele. Restaurants offer QR codes to their customers by which they can answer a couple of questions about their server. And, at the end of customer support calls, companies invite customers to press a number to rate the person they interacted with.
CSAT questions generally focus on understanding the experience immediately at hand:
- Are you satisfied or unsatisfied with the customer support you just received?
- How would you rate the quality of the service you received today?
- How easy was it to use our website?
- What could we have done better today?
CSAT can also be measured via qualitative coding and sentiment analysis of online reviews, social media comments, and customer support chats. With the help of AI, discrete qualitative comments can be quickly and easily coded into categories and themes to understand which areas drive customer satisfaction.
Customer Experience (CX) for Broad, Long-term Perceptions
Rather than focusing on a single customer touchpoint, customer experience research focuses on broader, longer-term relationships. For example, companies like Apple, Amazon, Disney, and Starbucks have earned reputations for creating painless omnichannel customer journeys. Regardless of whether people interact in-person with their customer service team, digitally through their website or apps, or physically through stores and product packaging, generations of customers have enjoyed positive experiences over multiple touchpoints and display high levels of customer loyalty and retention.
By measuring customer experience, brands can identify and resolve friction points throughout an omnichannel customer journey and improve the broader customer experience. Further, CSAT information can be used to prioritize the innovation of new products, tailor messaging for diverse target audiences, and make wise financial and strategic decisions about the business.
Because customer experience includes such a broad domain, it is often measured using a variety of quantitative questionnaires as well as qualitative interviews, focus groups, bulletin-boards, and open-end survey responses analyzed with AI. Possible questions include:
- On a scale from very satisfied to very unsatisfied, how would you rate your overall experience with us?
- On a scale from 0 to 10, how likely are you to recommend us to others? (NPS)
- Please describe your experience of working with our customer support team.
- How easy was it to start using our product?
- How intuitive is our product to use?
- What words would you use to describe our company?
- What would make you consider switching to another company?
Customer Success for Long-term Loyalty and Retention
While understanding both customer satisfaction and customer experience is critical, businesses also need to proactively prioritize customer success. Thus, instead of focusing only on delivering positive interactions, brands should work to ensure their products or services help clients achieve their long-term goals. The end result is long-term partnerships, increased customer lifetime value, and opportunities to unlock shared growth.
Because of this focus on shared growth, customer success is usually measured both quantitatively for tracking purposes and qualitatively to gather deep insights. In addition to incorporating customer satisfaction and customer experience questions, research into customer success may also include questions like:
- How well do our services help you achieve your goals?
- What kinds of tasks allow you to use our product to its full potential?
- What types of measurable value have you seen from using our services?
- How well does our customer support team provide you with the guidance you need?
- Does our team proactively help you maximize your use of our product?
- How effective was the training you received from our support team?
Integrating CX, CSAT, & Customer Success
Focusing on customer satisfaction is a quick and easy way to identify isolated weaknesses requiring resolution. However, to drive sustainable, long-term business growth, companies need to transform ad hoc customer satisfaction research projects into more comprehensive and customer experience and success programs.
With careful attention, this transformation can lead to the creation of research and activation feedback cycles that improve customer retention, cultivate brand advocates, and ultimately accelerate business growth.
The Voxco team prides itself on creating superior customer experiences and helping our partners find greater customer success. If you’d like to build a tailored customer experience and success research program, please get in touch with one of our helpful customer experience experts today!
Read more
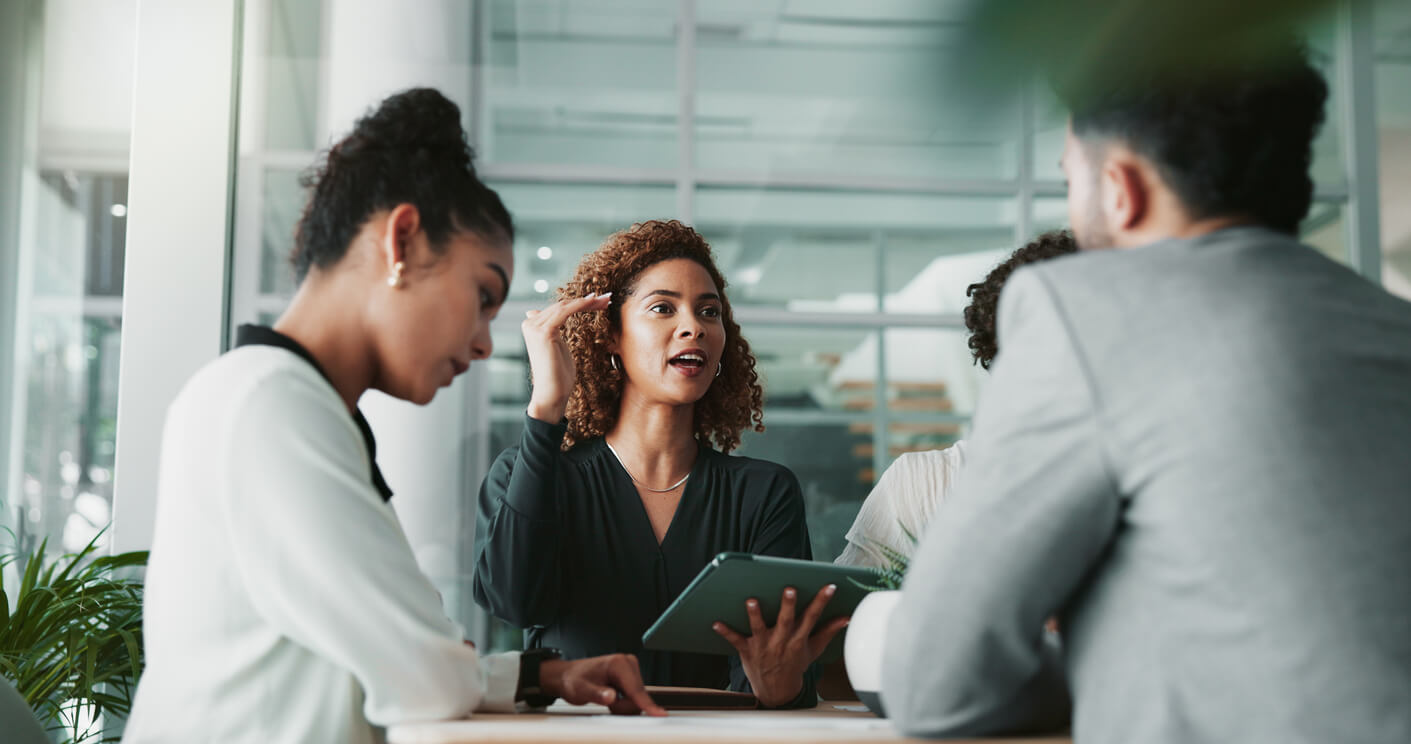
The Latest in Market Research
Becoming a Research Consultant: Skills, Mindset & Strategic Value
One of the key differences between early and later career researchers is their approach to working with clients. Many of us start off as traditional researchers and, over time, learn to become more consultative. But understanding and growing into a consultative style early on leads to advantages for both researchers and clients.
What questions do researchers and research consultants ask?
Traditional marketing researchers tend to have a laser focus on the data. Through rigorous and systematic techniques, they gather, analyze, and present data that generates essential knowledge to answer specific business problems. They may ask and answer questions like:
- What is the most appropriate research design to measure this behavior?
- How can we reduce bias in a questionnaire studying income disparities?
- What is the relationship between age and spending behaviors?
- Which theory is better supported by the evidence?
- Does X cause Y, and if so, how does it do so?
Research consultants also gather, analyze, and present data but their focus is broader and more strategic. They strive to develop deep insights that are forward-looking, opportunity driven, and improve business outcomes. They work to provide actionable recommendations and strategic roadmaps that serve the needs of executives and business decision-makers. Consultative researchers expand their focus beyond the data to include questions like:
- What is the most effective strategy to increase sales over the next 5 years?
- Why is the business losing market share and how can we reverse this trend?
- Which of these three strategies will yield the highest ROI in the short-term vs the long-term?
- Does future-proofing our business mean expanding into a new category or a new market?
- Which strategy will improve adult literacy and numeracy more quickly?
What Skills do Researchers and Research Consultants Have?
Both traditional and consultative market researchers must master essential core competencies to be effective in their roles. Along with a foundation of strong methodological expertise, both types of researchers have a solid understanding of professional standards and ethical guidelines developed by industry associations like Insights Association, CRIC, and ESOMAR. Specific skills include:
- Designing clear and unbiased surveys and discussion guides
- Quantitative proficiency to understand how, when, and why to use CATI, questionnaires, and analytics
- Qualitative proficiency to interview people, moderate groups, and perform content and sentiment analysis
- Using data to answer business and research questions with verifiable facts
- Preparing easy to read and understand reports and dashboards
However, to excel as a research consultant, an additional set of business and stakeholder management skills is essential. As such, research consultants expand their skill sets with bolder, subjective soft skills such as:
- Challenging requests and recommending unexpected solutions
- Bridging the gap between data collection and business impact
- Shaping organizational strategy by positioning themselves as partners
- Demonstrating proactive problem-solving abilities and anticipating client needs
- Mastering storytelling and persuasion to create compelling narratives that drive action
When to be Traditional VS Consultative
Some researchers love the methodological and systemic processes of creating and digging into data. On the other hand, some researchers love transforming data into long-term business strategies. Of course, just because you fall into the first category doesn’t mean you must automatically transition into the second.
Traditional market research roles are essential for large-scale projects employing standardized methodologies within established research teams. Where structured processes and clearly defined scopes guide the work, traditional roles ensure research results are reliable and consistent. Once you realize you’re a traditional researcher at heart, you’ll see that you are the backbone of insights generation.
On the other hand, research consultants shine when insights are necessary to guide strategic initiatives, especially when working with collaborative clients and cross-functional teams. This style suits researchers who want to be personally involved in shaping business decisions with long-term impacts.
Researchers who evolve into the consultant role develop a mindset of consistently asking “so what” and “now what.” They push beyond surface-level findings and uncover the real business implications of data. They engage stakeholders during the problem-definition phase rather than just the execution stage. They craft compelling narratives that connect data points to business outcomes rather than simply presenting spreadsheets and charts. It takes deliberate practice to evolve from being a number-crunchers to a strategic advisor but it’s a highly valued skill that builds personal gratification and loyalty from clients.
Seize the moment!
As a research buyer, you probably have a lot of experience working with traditional market researchers. Indeed, they are the powerhouse behind most major research projects. However, given our increasingly global economy, leveraging research consultants as strategic partners can help bridge the gap between data and strategy leading to long-term business growth.
At Voxco, we’ve partnered with lots of companies to help them achieve success with our CATI/AVR, survey, and text analytics research tools. We’d love to also partner with you to ensure you uncover the insights that matter most to your business. Please get in touch with one of our strategic research consultants.
Read more
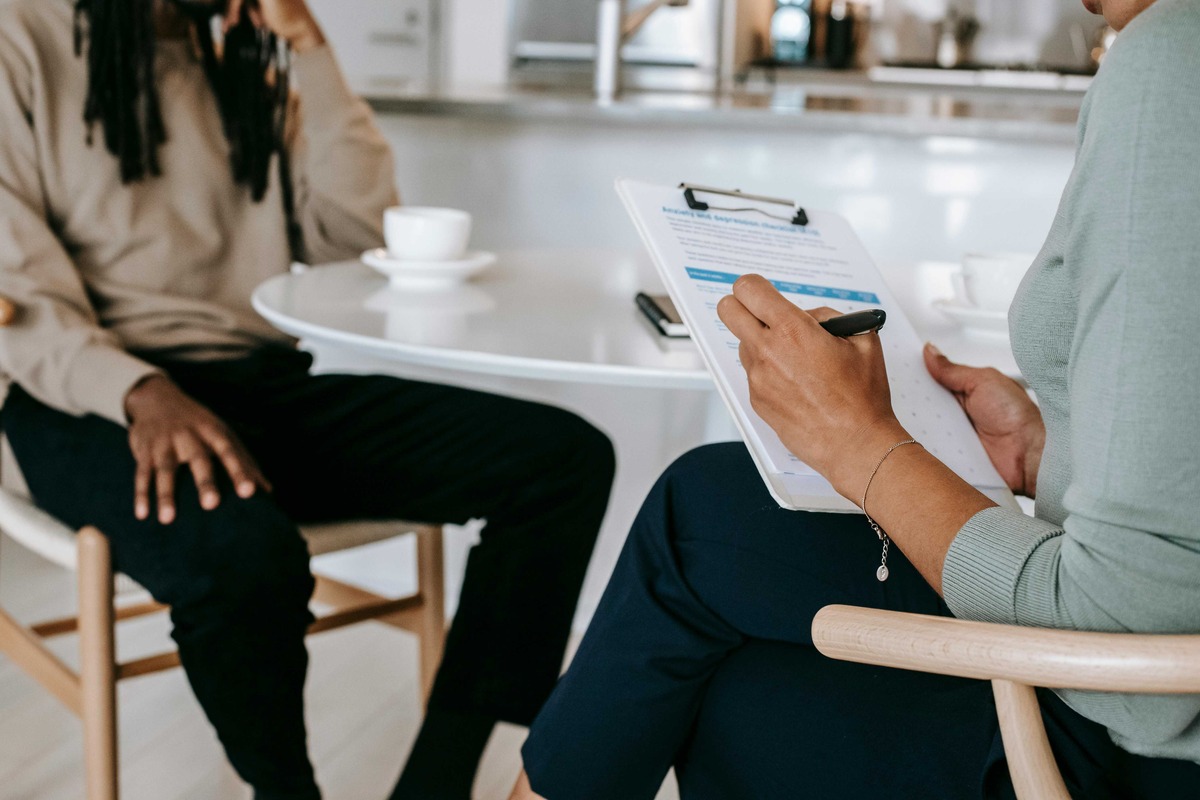
The Latest in Market Research
Becoming a Quallie: From factor analysis to AI qualitative coding
For social or market researchers who have years or decades of experience with quantitative data analysis, AI qualitative data analysis can feel intimidating. Researchers often specialize in quant or qual because the skill sets are quite different. However, you’ll soon see that qualitative data analysis has much in common with quantitative data analysis, especially if your experience is with advanced statistics like factor analysis or cluster analysis. Let’s find out why!
The qualitative side of factor and cluster analysis
When conducting a factor analysis, statistical software determines which answers tend to co-occur. For example, correlations will show that someone who indicates their job title is CEO or President probably also indicates they have a high income. Correlations will also show that someone who indicates they love bargains probably seeks out coupons and BOGOs. Across 100 questions, it’s impossible for a researcher to identify all the meaningful correlations among two, three, or ten variables.
That’s why we love when statistical software steps in with factor and cluster analysis. This software makes it much faster and easier to identify significant correlations and categorize 100 variables and 500 possible answers into several solutions, each one with 4, 5, 6, 7, 8, or 15 buckets. None of these solutions are inherently truth but as you review each set of buckets, you’ll generate a subjective opinion that one solution feels more valid and reliable than others. That solution will have:
- Fewer variables and answers that don’t seem to logically belong to the bucket they’ve been assigned to.
- Fewer buckets that look like a random collection of answers.
- More buckets that feel like cohesive, meaningful, nameable constructs.
After reviewing the various solutions, you’ll choose just one to name and claim. Despite the extensive amount of quantitative statistical analysis taking place, you can see that the final process is very qualitative.
The quantitative side of AI qualitative coding
Thirty years ago, before automated and generative AI tools simplified and sped up the process in a meaningful way, people did all the work manually. Over days and weeks, researchers would read paper questionnaires and transcripts to identify potential trends in the words and phrases and then determine whether there were broader connections and patterns among them. As technology improved, it became much easier and faster to search for concepts and assign qualitative codes (e.g., gender, anger, pricing) and quantitative codes (e.g., 1=Female, 7.2=Anger.High, 32=Pricing). Today, with the assistance of AI qualitative coding, creating a coding structure with multi-level netting and extracting descriptive themes from open end comments has turned months, weeks, and days into mere minutes.
A researcher with lots of qualitative experience might prefer to review and analyze the qualitative codes manually to generate possible theories and choose the best one. Similarly, someone with lots of quantitative experience might see envision the quantitative codes serving as a dataset for running a factor analysis. Whether you see the process from a qualitative or quantitative point of view, the better method of identifying a theory is the one that feels right to you and results in valid and actionable results.
Putting a human into the coding
Whether generated by AI or by human, having a set of codes and themes, named or not, does not mean the qualitative analysis is done. The next step is to review and understand the codes to identify a theory or result that is the most meaningful, strategic, and actionable.
- Which solution connects in a logical way with an existing consumer, personality, social, or economic theory?
- What aspects of the existing theory are currently missing from the analysis? Are those aspects still in the data or were they never a part of the data - why?
- What aspects of potential solution are different from the existing theory? Is the theory wrong? Is the solution wrong? What are the known or unknown caveats?
- Do the results warrant development of a new theory that will require additional research?
AI and statistical software don’t have the personal, social, emotional, psychological, and cultural understanding of the human experience - yet. What makes sense statistically will still need some tweaking to flush out the full depth of the human experience. That’s what expert market and social researchers bring to the table.
Summary
AI is no longer the wave of the future. It is today and it is in everything we do. From pattern recognition, theory development, logical reasoning, consumer behavior, and more, your skills as a quantitative researcher transfer in meaningful ways to the qualitative world. Consider AI qualitative coding as a slightly different way to conduct a factor or cluster analysis, a technique you already have fun with!
If you’d like to learn more about our AI tools, check out our AI qualitative coding tool, ascribe, and read Voxco’s answers to ESOMAR’S 20 Questions to Help Buyers of AI-Based Services. When you’re ready to speed up your qualitative data analysis with a one-stop tool, please get in touch with one of our survey experts.
Read more
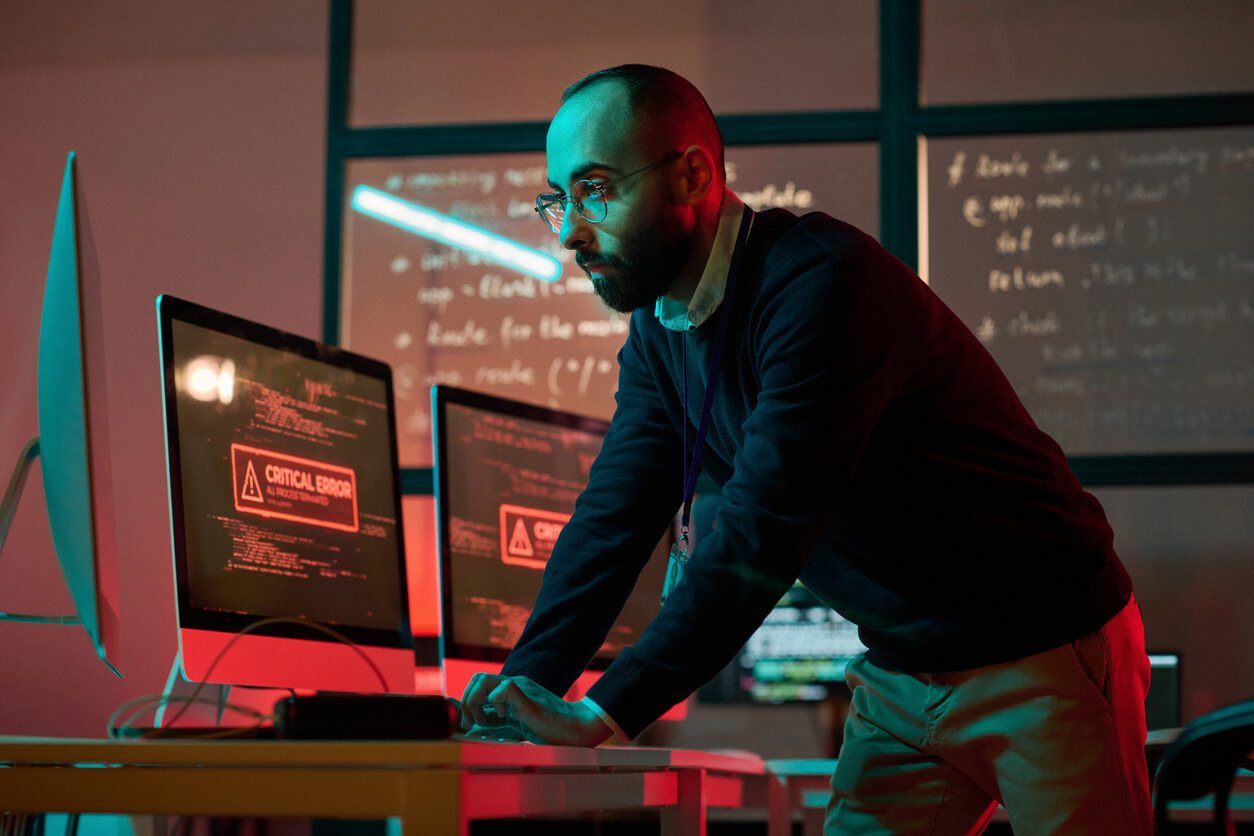
Market Research 101
Error to Insight: A Researcher’s Secret Weapon
We may not like to admit it but making mistakes is an expected part of the scientific process. As market and social researchers, we regularly discover errors, learn from them, and improve our processes because of them. You might even say that errors are essential for innovating and generating better insights. From the initial stages of data collection to later stages of results presentation, embracing mistakes is a pathway to better research.
Champion Methodological Mistakes
Plenty of errors are likely to occur during the research design and data collection phase. They reveal unexpected gaps that can be anticipated and prevented in future projects with better training, tools, and processes. How can we embrace these errors?
- Expect flawed data collection tools. Whether you’re working with discussion guides, questionnaires, diaries, or bulletin board outlines, errors such as leading questions, unclear scales, and missing response options are bound to appear. Plan for these problems by including time in the schedule for colleagues to review your tools, perhaps adding incentives for creative solutions. Further, be sure to pilot test the final tool with a few participants prior to the full launch.
- Train for neutrality. As hard as we try to avoid it, questionnaire authors, interviewers, and moderators have feelings and opinions that show up as biased questions, micro-expressions/body language, and tone of voice. This unintentional lack of neutrality can decrease participation rates and distort results. To address this problem, attend refresher training courses on a regular basis. You’ll not only be reminded of common mistakes you may have forgotten about but you’ll also learn new techniques that have gained prominence since your last training.
- Plan for sampling gaps. Every researcher knows how easy it is to recruit a large group of research participants. At the same time, every researcher also knows how difficult it is to recruit participants who are representative of the target population. When budget and field dates are pressuring you, avoid the temptation to overlook representativeness. Build extra time into the schedule and actively oversample difficult target audiences from the beginning to avoid the biases that will result from settling on non-representative samples.
Embrace Analytical Errors
Once data has been collected, the potential for errors doesn’t stop. By embracing errors during the analytical phase, we can achieve more thorough and nuanced data analysis.
- Seek outliers. Sometimes, outliers are discovered to be mistakes like miscodes or shifted columns. These are easily corrected. However, other outliers are analytical puzzles that need to be deciphered. It’s easy to automatically dismiss statistical anomalies but first consider whether they signal an unexpected insight. Mark the anomaly so that once you have a better understanding of the entire research problem, you can go back to it and determine if it was more meaningful than first realized.
- Contradict yourself. As you’re reviewing the analysis and building theories, actively contradict your own ideas. Try to prove the alternative hypothesis. Ask yourself what is missing. Assume errors have been made. Consider if you’ve overgeneralized beyond what the data is telling you. This will help you to avoid the confirmation bias that can arise out of early discoveries.
- Encourage dissent. Throughout your analysis, invite other team members to independently interpret your data. Incentivize them to contradict your interpretations and conclusions with plausible alternatives. And be prepared to put your ideas aside when other ideas are more likely.
- Leverage technology. Rushing through every potentially important theoretical model or statistical analysis is a fast-track to making errors. Use AI tools to dramatically improve your productivity and accuracy. Read our case studies to learn how C+R Research and Frost & Sullivan use Ascribe and Voxco Online to build complex questionnaires and code qualitative data faster, more accurately, and at a lower cost.
Capitalize on Communication Glitches
In many cases, stakeholders review research reports without guidance from the researcher. Consequently, it’s essential that reports are clear, engaging, and powerful.
- Test reports with stakeholders. After spending days and weeks writing a report, your headlines and summaries will seem clear and concise – to you. Schedule sufficient time into your plan so that non-researchers can review it for clarity. Invite them to interpret and mis-interpret charts and tables, and point out conclusions that don’t make sense. Incentives are a great idea here too as many people don’t feel comfortable sharing criticisms of their colleagues’ work.
- Use plain language. As much as you love talking about various statistical tests and study designs with your colleagues, research jargon is not plain language and will not help readers who are further down the chain. Yes, share the jargon as learning opportunities but also incorporate sufficient descriptions so that people who don’t know your jargon will still understand what you’re talking about.
- Highlight limitations. Limitations are not flaws or mistakes. They are acknowledgements that research can never uncover every possible insight regarding every possible scenario. Since no one knows the limitations of the research better than you do, share details about any study constraints during verbal presentations and in the report. Preventing misunderstandings is a key part of your role and your clients will appreciate the guardrails.
Convert Errors into Enhancements
Even the best researchers can’t avoid mistakes. What they do, however, is implement processes to anticipate, detect, and learn from those mistakes. Leverage AI and automation to reduce errors arising out of tedious activities. Collaborate with colleagues and other teams to invite dissenting opinions and alternative insights. Formalize debriefing sessions to identify problems and implement processes to prevent them in future projects.
Remember, mistakes aren’t failures. They’re feedback. By normalizing the presence of errors and fostering a culture of feedback, researchers can improve research validity and methodologies, build trust with stakeholders, and produce more impactful results. Embrace imperfection and the continuous improvement that goes along with it!
If you’d like to work with an experienced team of researchers who also value continual improvement, please get in touch with one of our research experts.
Read more
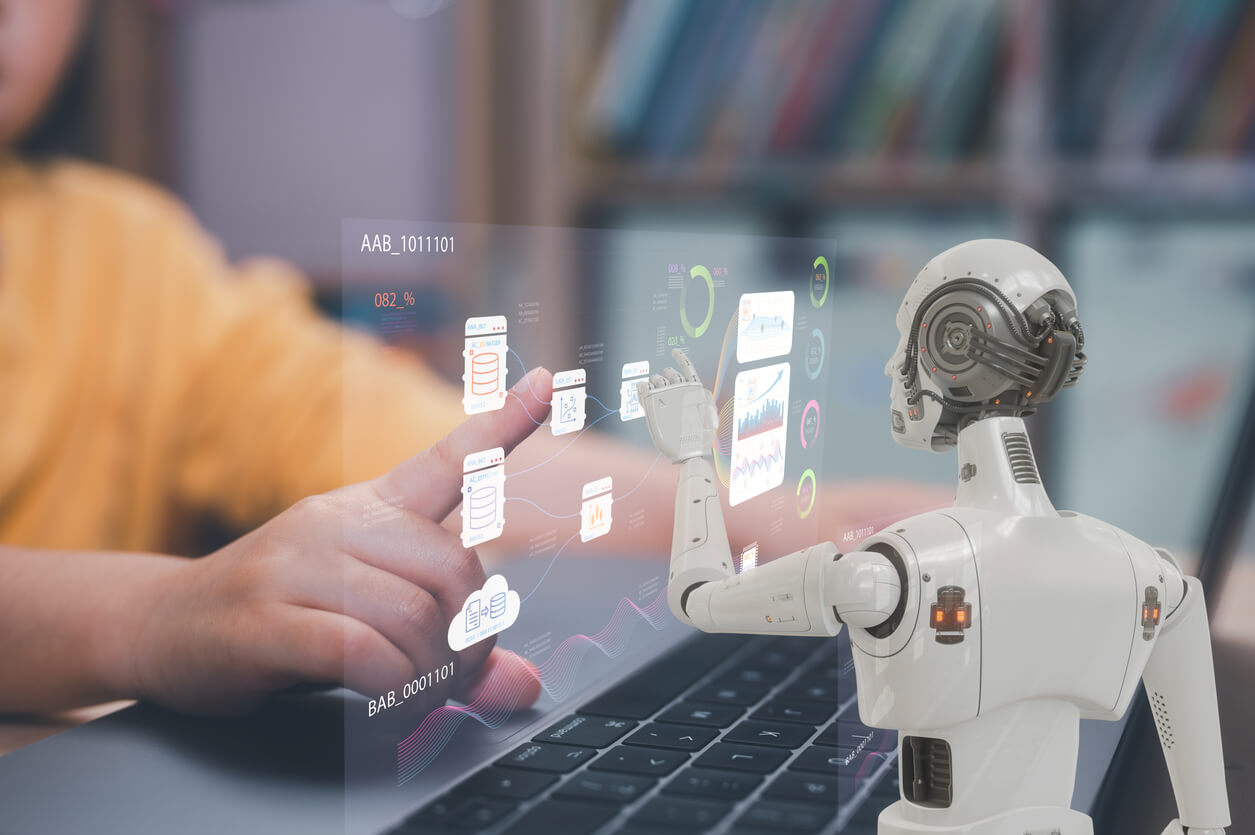
Text Analytics & AI
How to Choose the Right Solution
Why Ascribe Coder is Better Than ChatGPT at Analyzing Open-Ended Responses
In the age of AI, analyzing open-ended survey responses is more efficient than ever. While general AI models like ChatGPT can process text, they lack the precision, structure, and market research (MR) expertise needed for accurate coding and categorization. Ascribe Coder stands out as the superior choice for researchers, offering a purpose-built solution designed specifically for open-end analysis. Here’s why:
Purpose-Built for Market Research & Survey Analysis
Unlike ChatGPT, which is a general-purpose language model, Ascribe Coder is specifically designed to process, categorize, and analyze open-ended responses. It employs a proprietary method for extracting themes, refined over decades of market research experience. This specialization ensures that responses are coded meaningfully, aligning with industry expectations.
Consistent, Reliable, and Transparent Coding
One of the major drawbacks of ChatGPT is the potential for inconsistent responses due to its generative nature. Ascribe Coder, on the other hand, provides:
- Structured and reproducible coding, with clear parameters and guardrails to keep results focused on the topic.
- Transparency, in the process ensures applied codes stay linked to the original context and meaning within responses.
- Partially coded response reporting, making it easy to review and refine results.
Customizable Codebooks
Ascribe Coder gives users complete control over the codebook or code frame. Researchers can:
- Automatically create descriptive, thematic codebooks tailored to their projects and refine as needed.
- Create code frames with hierarchical and multi-level netting for detailed categorization.
- Save and reuse codebooks for ongoing or similar projects
This level of customization is not possible with ChatGPT, which lacks structured taxonomy management.
Scalability & Efficiency with AI Flexibility
Market researchers often handle large volumes of text data. Sometimes they prefer to use AI assistance for coding, and sometimes they prefer, or are required, not to use AI. Ascribe Coder is built for scalability and flexibility. It:
- Handles large volumes of text with fully automated, fully manual, or hybrid processing to fit user or project needs.
- Offers both generative and non-generative processing modes to suit different analysis needs
In contrast, ChatGPT processes text one query at a time, making it inefficient for large-scale analysis.
Seamless Integration with Market Research Workflows
Ascribe Coder fits seamlessly into research ecosystems, offering:
- Direct integration with survey platforms for smoother data processing.
- Access to Ascribe Coder’s project management system for improved workflow visibility and efficiency.
- Access to MR deliverables when needed for further analysis and reporting.
- Built-in visualization tools, delivering instant insights.
- ASK Ascribe, a tool allowing users to ask questions of the analysis and immediately receive insights, summaries and reports.
Human Oversight of Coding for AI Accuracy & Control
AI should enhance, not replace, human expertise. Ascribe Coder embraces human oversight, enabling researchers to:
- Supervise and customize AI-generated results and make adjustments as needed.
- Use integrated power tools to oversee, refine, and ensure quality control of AI coding.
ChatGPT, in contrast, operates autonomously, making it harder to systematically validate results.
Language Agnostic
Global research often requires analysis of multilingual responses. Ascribe Coder is language agnostic, meaning:
- It can create codebooks and present results in any language, regardless of the input language.
ChatGPT struggles with structured multilingual coding and lacks built-in tools for cross-language consistency.
Exceptional Customer Training and Support
Often times researchers need help with a complex project, training a new team member, or exporting results in a new format. Ascribe’s Customer Training and Support teams have worked for 20+ years in the business and are there to help when needed, ensuring customers receive the results they need from their data to deliver their business objectives. This makes Ascribe a true research companion, far beyond what ChatGPT can offer.
Conclusion
While ChatGPT is a powerful AI model, it was never designed for market research and survey coding. Ascribe Coder delivers structured, transparent, scalable, and customizable text analysis tailored to researchers’ needs. Its proprietary AI, seamless integrations and interactive tools all with human oversight, make it the superior choice for analyzing open-ended responses.
For researchers who demand accuracy, efficiency, and control, Ascribe Coder is the clear winner.
Read more
.jpg)
Market Research 101
Questionnaire Design Misconceptions: 6 Myths That Are Holding You Back
Whether you’re conducting market research, gathering customer feedback, or running an academic study, the quality of your questionnaire can make or break your results. Unfortunately, many people start the process with questionnaire misconceptions that lead to poorly designed surveys and sabotage their efforts before they even begin.
Whether you’re a seasoned researcher or a first-time questionnaire writer, understanding these pitfalls will help you design more effective, engaging, and accurate questionnaires. Let’s debunk the myths, avoid the mistakes, and get on the path to creating surveys that deliver meaningful insights.
Misconception #1: Anyone can write a questionnaire.
Despite how easy it looks, writing a high-quality questionnaire that generates valid and reliable data requires exceptional skill and experience. People are biased, fallible, and take shortcuts. Language is ambiguous, cultural, and plastic. Accommodating for people and language while translating a research objective into a set of questions that will be interpreted in a consistent way by most participants is a complex task. If you’re at the beginning of your questionnaire writing journey, partner with an experienced researcher who can guide and advise you along the way. The truth is, once you’ve gained sufficient expertise and mentorship, anyone can write a questionnaire.
Misconception #2: One size fits all.
Based on their personal experience and education, every researcher has a unique writing style that gets reflected their questionnaires. However, as much as you like your style, it’s not suited for every questionnaire. Younger people might prefer a more gamified survey. People who read English as a 2nd, 3rd, or 4th language might not understand the slang, expressions, idioms, and jargon that are second nature for you. Questionnaires that focus on sensitive issues need empathetic and gentle language. Before writing any questionnaire, researchers must think about their target audience and adjust their writing style to be appropriate for that audience.
Misconception #3: Any question will do.
In many cases, there are unnumerable ways to ask any single question and not one of them is perfect. Single-selects, multi-selects, rankings, ratings, open ends – all these question types could be suitable to ask any consumer, customer, and social behaviors and opinions. However, with experience, you’ll learn that each question type engenders different types of error and interpretations. By choosing a question type, you are also choosing which type of error you are willing to accept. Once you’ve decided what kind of data you need and which errors you can work with, the best questions are clear and concise.
Misconception #4: There is a perfect set of answers.
Just as there are no perfect questions, there are also no perfect answer sets. It’s impossible to say that 5 or 7 or 10 options is the correct number of options. And, it’s impossible to say which set of numbers, brands, or concepts should be included in the set. At some point, you must exclude answers that some people would want to choose in order to create a better experience for the majority of participants. Make sure you’ve got an effective opt-out in place (e.g., Other, Not applicable, None of the above) and then move on.
Misconception #5: People are perfect.
As much as we want participants to read and answer every question perfectly, it’s not going to happen. Researchers rely on sample companies to remove large scale fraud so when we see evidence of that, it’s important to report it back to the company. On the other hand, when we can identify people who are bored, tired, distracted, and not reading carefully, we need to remember that this is normal, human behavior. It’s the researcher’s responsibility to design questionnaires that identify and set aside participants who aren’t having a good day today.
Misconception #6: Questionnaires are boring.
I’m glad to say that questionnaires USED to be boring. Now, we have an abundance of question formats to choose from, including imagery, audio, videos, and gamification. When combined with engaging, fun, and interesting wording, questionnaires never have to be boring again.
Summary
Designing questionnaires is a perfect example of how tasks that appear simple are ultimately revealed to be complex once you dive in. As such, even the best questionnaire designers constantly seek to improve their work. They may seek advice from other experienced researchers, read new questionnaire design books, or take a course to sharpen their skills. Many survey platforms also offer pre-tested, templated questions that have been pre-tested to reduce bias and ensure high-quality results. If you’re seeking some expert guidance, please reach out to one of our survey experts today!
Read more

Market Research 101
Research Participant Rights 101: What Every Social and Market Researcher Needs to Understand
Research guidelines and standards tend to focus on the ideal methodological practices that social and market researchers need to undertake. Though that focus is extremely important, it’s also important to consider research from the point of view of the people who voluntarily take time out of their day to share their thoughts and opinions. Here are 7 core participant rights that researchers should accommodate in any research they conduct.
Consent
“Informed consent’ may be a more popular term but, if you think about it, it is impossible to give consent without first being fully informed. Consent is the entire requirement. Research participants have the right to be informed about a variety of things including:
- General topic and purpose of the research. Exceptions would include where doing so would invalidate the research results. In such cases, the topic and purpose could be revealed after the study is complete.
- The company conducting the research and the name of a person who can answer their questions.
- How their information will be used
- Whether any video or audio recordings will be made
- How much time is required
- What incentives will be offered
- Risks and benefits to their participation
What does this mean for researchers?
Beyond ensuring you share as many details as possible in research invitations, be sure a single contact person is prepared to answer questions posed by potential or current participants. And, ensure that person is easy to reach – participants should not have to send multiple emails or navigate multiple hyperlinks to access them. The onus is on the research company to ensure participants reach that person within one or two efforts.
Voluntary Participation and Withdrawal
Research participants have the right to withdraw their participation at any moment guiltfree and without penalty. This includes not participating in portions or the remainder of a study.
What does this mean for researchers?
While researchers can confirm that a participant wishes to skip a section of or halt the entire study, they may not intimidate or try to coerce participants into continuing. Things could get uncomfortable in a group setting but it’s up to the researcher to ensure the departing participant leaves with grace.
Confidentiality and Privacy
Research participants have the right to control their own information. This includes determining whether and with whom their personal information may be shared, and to have their personal information removed at any point. Participants also have the right to receive a research supplier’s privacy policy with ease and in a reasonable time frame.
What does this mean for researchers?
Most importantly, researchers should ensure their privacy policy is easily available on their website (here’s ours), and can be shared as a hyperlink in email invitations or follow up messaging. Additionally, research companies should have a formal process in place by which a participant’s information can be quickly removed.
Anonymization
Unless they have given consent (which we now know means they have all relevant information), research participants have the right for their information to be fully anonymized.
What does this mean for researchers?
When sharing verbatims in reports, change the details of the individuals involved. Mary becomes Susan, Mohommed become Hussain, Khari becomes Kanan. Even better, age 27 becomes age 29 and age 47 becomes age 46. Further, typos and language style can be preserved while also being altered. Thus, “you aint gonna do nothing” can become “you not gone do nothing.” As researchers, our goal is to preserve concepts, styles, and opinions not make it easy to identify the only woman at the local library who uses the word ‘ain’t.’
Safety
Safety is about more than preventing burnt tongues during a sensory test of soup or preventing cut fingers during a test of an innovative knife handle. Research participants also have the right to know about potential mental or emotional harm that may arise from feeling judged or shamed during discussions of sensitive topics like income, employment, health, sexuality, disabilities, religion, and children.
What does this mean for researchers?
Especially in the case of person-to-person research (e.g., focus groups, individual interviews, telephone interviews), ensure that precautions are taken so people don’t feel embarrassed or harassed by your questions. And, be sure to tell people at the beginning of the study if the topic or any questions could be perceived as sensitive.
Respect
Every research participant, regardless of age, gender, sexuality, disability, religion, and ethnicity, has the right to be treated respectfully during all stages of the research process.
What does this mean for researchers?
Respect plays out in many ways beyond respectful words and communications. As an overlying requirement, researchers must look out for and advocate for participant rights. More specifically, it includes asking only those questions that will actually be analyzed, being honest about how long participation will be, keeping screeners as short as possible, using advanced skip logic and piping to keep surveys short and readable, and ensuring your data collections tools are inclusive. Think about when you’re standing at a customer service desk at a store getting annoyed – this is what you are aiming to prevent with your research experience.
Research Only
Finally, research participants have the right to not be part of bait and switch tactics, whether mugging (marketing under the guide of research), sugging (selling…), or frugging (fund raising…).
What does this mean for researchers?
If you aren’t genuinely conducting research, don’t mislead people into thinking you are. That includes not using PII for direct marketing, list-building or any activities that aren’t directly related to the study and for which consent was not given. If you hope to offer special deals to people at the end of your survey, you don’t need research sample. You need an email or direct marketing list. Don’t damage the reputation of the market and social research industry by misusing research sample.
Summary
Though we’ve identified 7 core participants rights, they could be encapsulated under one single right – the right to be treated with respect. Respect means sharing information, respecting privacy, enforcing anonymity, not misleading people, and listening to people’s needs. By prioritizing respondent rights, market researchers not only comply with ethical and legal standards, they also foster engaged participation, leading to more accurate insights and long-term success. If you’re curious to learn more about our standards of research practice, please get in touch with one of our survey experts.
Additional Resources for Research Participants Rights
- Insights Association Participant Bill of Rights
- ESOMAR/WAPOR GUIDELINE ON OPINION POLLS AND PUBLISHED SURVEYS
- CRIC Public Opinion Research Standards and Disclosure Requirements
- MRIA Charter of Respondent Rights (MRIA no longer exists but the information remains important)
- Standards for the Conduct of Government of Canada Public Opinion Research
Read more