Written by
What is Inferential Statistics?
Inferential Statistics in quantitative research works in addition to Descriptive Statistics. Where descriptive statistics helps to summarize the characteristics of a sample population, inferential statistics focuses on using that summarized data and predict the characteristics for the larger population.

Hence, inferential statistics allows a researcher to make assumptions based on small populations, for the bigger populations. It uses measurements from the sample group and makes generalizations based on them for the larger groups. In essence to what is being said, if you want to identify whether the current statistics are descriptive or inferential, the key is that descriptive statistics works on your current sample data set and inference statistics work on concluding data in addition to your sample dataset which is comparatively huge.Example:You want to know the occupations of people in a village. You collect sample data from diverse villagers. After performing descriptive statistics on it, inferential statistics will give you an idea about what all occupations the rest of the villagers must be into.
What are the problems faced while collecting samples in Inferential Statistics?
- You have to make sure the sample data you select is diverse and random. This will avoid the results being biased. The sample data needs to be representative of the entire population for your assumptions to be accurate.
- Errors in sampling can happen when you don’t cover some data from the population in your data. It creates a difference between the actual values of the population and the values that you have measured. This can happen irrespective of the first point. Nevertheless, there are various probability sampling methods to help avoid this like Systematic sampling, Cluster sampling, stratified sampling as Simple Random sampling.
Ways of estimating population parameters from sample statistics:
It ideally tells us how to convert descriptive statistics to inference statistics. As the heading suggests, there are two characteristics:
- Parameter- Describes the entire population (mean of the population).
- Statistic- Describes the sample data (mean of the sample).
Inference statistics help us to use the sample statistic and describe the population parameter by taking sampling error into account and minimizing the difference between the two.
Types of estimating the population:
- Point Estimate- It uses the sample data to calculate a single value or point, a statistic that is the best estimate of a population parameter. It identifies a point in some parameter space.
- Interval Estimate- Unlike point estimate, interval estimate gives a range where the parameter is expected to belong to.
- Confidence Interval: Most common type of use of Interval Estimate. It is a range of values where the estimates are expected to fall on running the experiment again or on re-sampling. Hence, it is the mean of estimates which can fall on either on plus side or minus side of the variance (upper and lower bounds). Confidence level states the percentage of times we can expect the value to fall between the bounds. More the confidence level, the more the estimates are likely to fall in the same upper and lower bounds of the confidence interval.
What is Hypothesis Testing in Inferential Statistics?
It is a statistically followed process to testing the assumptions made depending on The morris sample data. Let’s take an example along with the five steps to be followed while performing Hypothesis Testing;
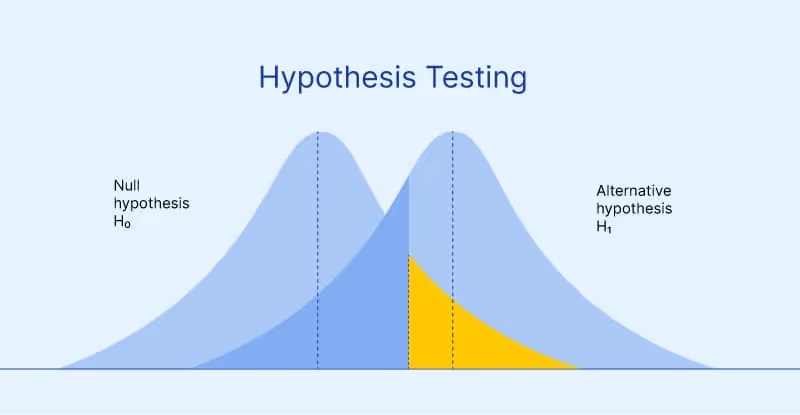
Step 1) State Null hypothesis (H0):
It is an already accepted fact. Analysts try to eliminate or nullify the null hypothesis.
Step 2) State the Alternate hypothesis (Ha):
It the opposite of the null hypothesis and analysts try statistically to prove the alternate hypothesis right. If it is proved that the alternate hypothesis is right then the null hypothesis is automatically rejected. And vice versa.
Step 3) Determine Significance Level (α):
It is a determiner that states the probability of the alternate hypothesis being right. It uses a significance level which tells how confident you are in your conclusion. Generally it is 0.05 (5%) to start with. If α=0.05, then it will mean that you can 5% support the alternate hypothesis (meaning reject the null hypothesis). But it would mean that you were wrong to reject the null hypothesis.
Step 4) Calculate p-value:
It is a probability value in favour of the null hypothesis. Significance level supports the alternate hypothesis, p-value supports the null hypothesis.
- High significance level -> alternate hypothesis (null hypothesis rejected)
- High p-value-> null hypothesis (alternate hypothesis rejected)Step 5) Conclusion:
On basis of the above conditions, you can accept or reject the hypothesis by looking at the percentage of p-value and significance level.Example:(H0)- Obese people are insecure about their weight.(Ha)- Obese people don’t let their weight pull down their self-confidence. (α)- 0.05P-value- 0.02Conclusion- on interviewing several obese people you found out that many people are body positive and are comfortable in their skin. P-value is 0.02 which is way low than the significance level of 0.05, you failed to prove the null hypothesis and thereby accept the alternate hypothesis saying obese people don’t let their weight pull down their self-confidence.
What are Statistical Tests in Inferential Statistics?
Useful to test out predictions and hypotheses, it also eradicates sampling errors.Parametric statistical tests- more powerful due to their defect detection. It does so by making assumptions regarding the normal distribution of scores, the definition of the population by sample data, etc.Non-parametric statistical tests- do not make any assumptions and are distribution-free tests.
What are the types of Statistical Tests in Inferential Statistics?
Comparison tests-
It looks for the differences in mean and medians or scores. To know which test approach will suit the aim, you need to check if your data meets the conditions for the respective tests.Type of Comparison testParametric?What’s being compared?Samplest-testYesMeans2 samplesANOVAYesMeans3+ samplesMood’s medianNoMedians2+ samplesWilcoxon signed-rankNoDistributions2 samplesWilcoxon rank-sum (Mann-WhitneyU)NoSums of rankings2 samplesKruskal-WallisHNoMean rankings3+ samples
Correlation tests-
Tell the level of association between two variables.Type of Correlation testParametric?VariablesPearson’srYesInterval/ratio variablesSpearman’s rNoOrdinal/interval/ratio variablesChi square test of independenceNoNominal/ordinal variables
Regression tests-
They show if you change the predicted variables whether it causes the output variables to change or not. Depending on the number of variables you have, you can decide whether to take the regression tests or not.Types of Regression testPredictorOutcomeSimple linear regression1 interval/ratio variable1 interval/ratio variableMultiple linear regression2+ interval/ratio variable(s)1 interval/ratio variableLogistic regression1+ any variable(s)1 binary variableNominal regression1+ any variable(s)1 nominal variableOrdinal regression1+ any variable(s)1 ordinal variable